Technical writing is hard to do well and it’s also a bit different from other types of writing. While good technical writing has no strict definition I do think there is a kind of ‘hierarchy of needs’ that defines it. I’m not sure this is complete or perfect but I find categorizing to be useful.
L1 – Writing Clearly
The author writes in a way that accurately represents the information they want to convey. Sentences have a clear purpose. The structure of the text flows from point to point.
L2 – Explaining Well (Logos in rhetorical theory)
The author breaks their argument down into logical blocks that build on one another to make complex ideas easier to understand. When done well, this almost always involves (a) short, inline examples to ground abstract ideas and (b) a concise and logical flow through the argument which does not repeat other than for grammatical effect or flip-flop between points.
L3 – Style
The author uses different turns of phrase, switches in person, different grammatical structures, humor, etc. to make their writing more interesting to read. Good style keeps the reader engaged. You know it when you see it as the ideas flow more easily into your mind. Really good style even evokes an emotion of its own. By contrast, an author can write clearly and explain well, but in a way that feels monotonous or even boring.
L4 – Evoking Emotion (Pathos in rhetorical theory)
I think this is the most advanced and also the most powerful particularly where it inspires the reader to take action based on your words through an emotional argument. To take an example, Martin Kleppmann’s turning the database inside out inspired a whole generation of software engineers to rethink how they build systems. Tim or Kris’ humor works in a different but equally effective way. Other appeals include establishing a connection with the reader, grounding in a subculture that the author and reader belong to, establishing credibility (ethos), highlighting where they are missing out on (FOMO), influencing through knowing and opinionated command of the content. There are many more.
The use of pathos (sadly) doesn’t always imply logos, often there are logical fallacies used even in technical writing. Writing is so much more powerful if both are used together.
This post provides a terse summary of the high-level arguments addressed in my book.
Why Change is Needed
Technology has changed:
- Partitioned/Replayable logs provide previously unattainable levels of throughput (up to Terabit/s), storage (up to PB) and high availability.
- Stateful Stream Processors include a rich suite of utilities for handling Streams, Tables, Joins, Buffering of late events (important in asynchronous communication), state management. These tools interface directly with business logic. Transactions tie streams and state together efficiently.
- Kafka Streams and KSQL are DSLs which can be run as standalone clusters, or embedded into applications and services directly. The latter approach makes streaming an API, interfacing inbound and outbound streams directly into your code.
Businesses need asynchronicity:
- Businesses are a collection of people, teams and departments performing a wide range of functions, backed by technology. Teams need to work asynchronously with respect to one another to be efficient.
- Many business processes are inherently asynchronous, for example shipping a parcel from a warehouse to a user’s door.
- A business may start as a website, where the front end makes synchronous calls to backend services, but as it grows the web of synchronous calls tightly couple services together at runtime. Event-based methods reverse this, decoupling systems in time and allowing them to evolve independently of one another.
A message broker has notable benefits:
- It flips control of routing, so a sender does not know who receives a message, and there may be many different receivers (pub/sub). This makes the system pluggable, as the producer is decoupled from the potentially many consumers.
- Load and scalability become a concern of the broker, not the source system.
- There is no requirement for backpressure. The receiver defines their own flow control.
Systems still require Request Response
- Whilst many systems are built entirely-event driven, request-response protocols remain the best choice for many use cases. The rule of thumb is: use request-response for intra-system communication particularly queries or lookups (customers, shopping carts, DNS), use events for state changes and inter-system communication (changes to business facts that are needed beyond the scope of the originating system).
Data-on-the-outside is different:
- In service-based ecosystems the data that services share is very different to the data they keep inside their service boundary. Outside data is harder to change, but it has more value in a holistic sense.
- The events services share form a journal, or ‘Shared Narrative’, describing exactly how your business evolved over time.
Databases aren’t well shared:
- Databases have rich interfaces that couple them tightly with the programs that use them. This makes them useful tools for data manipulation and storage, but poor tools for data integration.
- Shared databases form a bottleneck (performance, operability, storage etc.).
Data Services are still “databases”:
- A database wrapped in a service interface still suffers from many of the issues seen with shared databases (The Integration Database Antipattern). Either it provides all the functionality you need (becoming a homegrown database) or it provides a mechanism for extracting that data and moving it (becoming a homegrown replayable log).
Data movement is inevitable as ecosystems grow.
- The core datasets of any large business end up being distributed to the majority of applications.
- Messaging moves data from a tightly coupled place (the originating service) to a loosely coupled place (the service that is using the data). Because this gives teams more freedom (operationally, data enrichment, processing), it tends to be where they eventually end up.
Why Event Streaming
Events should be 1st Class Entities:
- Events are two things: (a) a notification and (b) a state transfer. The former leads to stateless architectures, the latter to stateful architectures. Both are useful.
- Events become a Shared Narrative describing the evolution of the business over time: When used with a replayable log, service interactions create a journal that describes everything a business does, one event at a time. This journal is useful for audit, replay (event sourcing) and debugging inter-service issues.
- Event-Driven Architectures move data to wherever it is needed: Traditional services are about isolating functionality that can be called upon and reused. Event-Driven architectures are about moving data to code, be it a different process, geography, disconnected device etc. Companies need both. The larger and more complex a system gets, the more it needs to replicate state.
Messaging is the most decoupled form of communication:
- Coupling relates to a combination of (a) data, (b) function and (c) operability
- Businesses have core datasets: these provide a base level of unavoidable coupling.
- Messaging moves this data from a highly coupled source to a loosely coupled destination which gives destination services control.
A Replayable Log turns ‘Ephemeral Messaging’ into ‘Messaging that Remembers’:
- Replayable logs can hold large, “Canonical” datasets where anyone can access them.
- You don’t ‘query’ a log in the traditional sense. You extract the data and create a view, in a cache or database of your own, or you process it in flight. The replayable log provides a central reference. This pattern gives each service the “slack” they need to iterate and change, as well as fitting the ‘derived view’ to the problem they need to solve.
Replayable Logs work better at keeping datasets in sync across a company:
- Data that is copied around a company can be hard to keep in sync. The different copies have a tendency to slowly diverge over time. Use of messaging in industry has highlighted this.
- If messaging ‘remembers’, it’s easier to stay in sync. The back-catalogue of data—the source of truth–is readily available.
- Streaming encourages derived views to be frequently re-derived. This keeps them close to the data in the log.
Replayable logs lead to Polyglot Views:
- There is no one-size-fits-all in data technology.
- Logs let you have many different data technologies, or data representations, sourced from the same place.
In Event-Driven Systems the Data Layer isn’t static
- In traditional applications the data layer is a database that is queried. In event-driven systems the data layer is a stream processor that prepares and coalesces data into a single event stream for ingest by a service or function.
- KSQL can be used as a data preparation layer that sits apart from the business functionality. KStreams can be used to embed the same functionality into a service.
- The streaming approach removes shared state (for example a database shared by different processes) allowing systems to scale without contention.
The ‘Database Inside Out’ analogy is useful when applied at cross-team or company scales:
- A streaming system can be thought of as a database turned inside out. A commit log and a a set of materialized views, caches and indexes created in different datastores or in the streaming system itself. This leads to two benefits.
- Data locality is used to increase performance: data is streamed to where it is needed, in a different application, a different geography, a different platform, etc.
- Data locality is used to increase autonomy: Each view can be controlled independently of the central log.
- At company scales this pattern works well because it carefully balances the need to centralize data (to keep it accurate), with the need to decentralise data access (to keep the organisation moving).
Streaming is a State of Mind:
- Databases, Request-response protocols and imperative programming lead us to think in blocking calls and command and control structures. Thinking of a business solely in this way is flawed.
- The streaming mindset starts by asking “what happens in the real world?” and “how does the real world evolve in time?” The business process is then modelled as a set of continuously computing functions driven by these real-world events.
- Request-response is about displaying information to users. Batch processing is about offline reporting. Streaming is about everything that happens in between.
The Streaming Way:
- Broadcast events
- Cache shared datasets in the log and make them discoverable.
- Let users manipulate event streams directly (e.g., with a streaming engine like KSQL)
- Drive simple microservices or FaaS, or create use-case-specific views in a database of your choice
The various points above lead to a set of broader principles that summarise the properties we expect in this type of system:
The WIRED Principles
Windowed: Reason accurately about an asynchronous world.
Immutable: Build on a replayable narrative of events.
Reactive: Be asynchronous, elastic & responsive.
Evolutionary: Decouple. Be pluggable. Use canonical event streams.
Data-Enabled: Move data to services and keep it in sync.
This post outlines how you might create a Request-Response Gateway in Kafka using the good old correlation ID trick and a shared response topic. It’s just a sketch. I haven’t tried it out.
A Rest Gateway provides an efficient Request-Response bridge to Kafka. This is in some ways a logical extension of the REST Proxy, wrapping the concepts of both a request and a response.
What problem does it solve?
- Allows you to contact a service, and get a response back, for example:
- to display the contents of the user’s shopping basket
- to validate and create a new order.
- Access many different services, with their implementation abstracted behind a topic name.
- Simple Restful interface removes the need for asynchronous programming front-side of the gateway.
So you may wonder: Why not simply expose a REST interface on a Service directly? The gateway lets you access many different services, and the topic abstraction provides a level of indirection in much the same way that service discovery does in a traditional request-response architecture. So backend services can be scaled out, instances taken down for maintenance etc, all behind the topic abstraction. In addition the Gateway can provide observability metrics etc in much the same way as a service mesh does.
You may also wonder: Do I really want to do request response in Kafka? For commands, which are typically business events that have a return value, there is a good argument for doing this in Kafka. The command is a business event and is typically something you want a record of. For queries it is different as there is little benefit to using a broker, there is no need for broadcast and there is no need for retention, so this offers little value over a point-to-point interface like a HTTP request. So the latter case we wouldn’t recommend this approach over say HTTP, but it is still useful for advocates who want a single transport and value that over the redundancy of using a broker for request response (and yes these people exist).
This pattern can be extended to be a sidecar rather than a gateway also (although the number of response topics could potentially become an issue in an architecture with many sidecars).
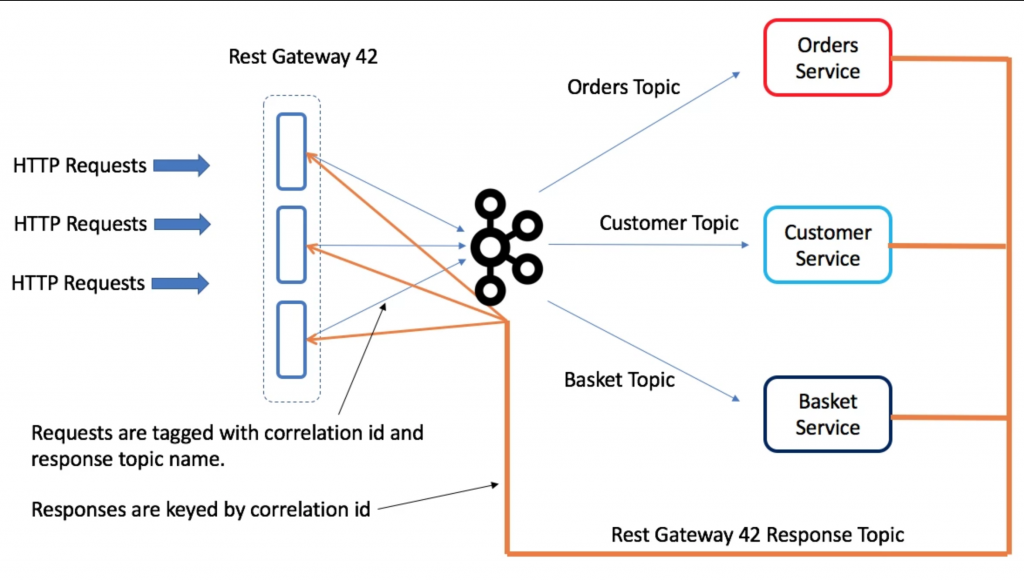
Implementation
Above we have a gateway running three instances, there are three services: Orders, Customer and Basket. Each service has a dedicated request topic that maps to that entity. There is a single response topic dedicated to the Gateway.
The gateway is configured to support different services, each taking 1 request topic and 1 response topic.
Imagine we POST and Order and expect confirmation back from the Orders service that it was saved. This work as follows:
- The HTTP request arrives at one node in the Gateway. It is assigned a correlation ID.
- The correlation ID is derived so that it hashes to a partition of the response topic owned by this gateway node (we need this to route the request back to the correct instance). Alternatively a random correlation id could be assigned and the request forwarded to the gateway node that owns the corresponding partition of the response topic.
- The request is tagged with a unique correlation ID and the name of the gateway response topic (each gateway has a dedicated response topic) then forwarded to the Orders Topic. The HTTP request is then parked in the webserver.
- The Orders Service processes the request and replies on the supplied response topic (i.e. the response topic of the REST Gateway), including the correlation ID as the key of the response message. When the REST Gateway receives the response, it extracts the correlation ID key and uses it to unblock the outstanding request so it responds to the user HTTP request.
Exactly the same process can be used for GET requests, although providing streaming GETs will require some form of batch markers or similar, which would be awkward for services to implement probably necessitating a client-side API.
If partitions move, whist requests are outstanding, they will timeout. We could work around this but it is likely acceptable for an initial version.
This is very similar to the way the OrdersService works in the Microservice Examples
Event-Driven Variant
When using an event driven architecture via event collaboration, responses aren’t based on a correlation id they are based on the event state, so for example we might submit orders, then respond once they are in a state of VALIDATED. The most common way to implement this is with CQRS.
Websocket Variant
Some users might prefer a websocket so that the response can trigger action rather than polling the gateway. Implementing a websocket interface is slightly more complex as you can’t use the queryable state API to redirect requests in the same way that you can with REST. There needs to be some table that maps (RequestId->Websocket(Client-Server)) which is used to ‘discover’ which node in the gateway has the websocket connection for some particular response.
The slides for the Craft Meetup can be found here.
I wrote a book: Designing Event Driven Systems
PDF
EPUB
MOBI (Kindle)

View full blogroll